Harnessing the Power of Data Labeling for Transformative Business Growth in Software Development
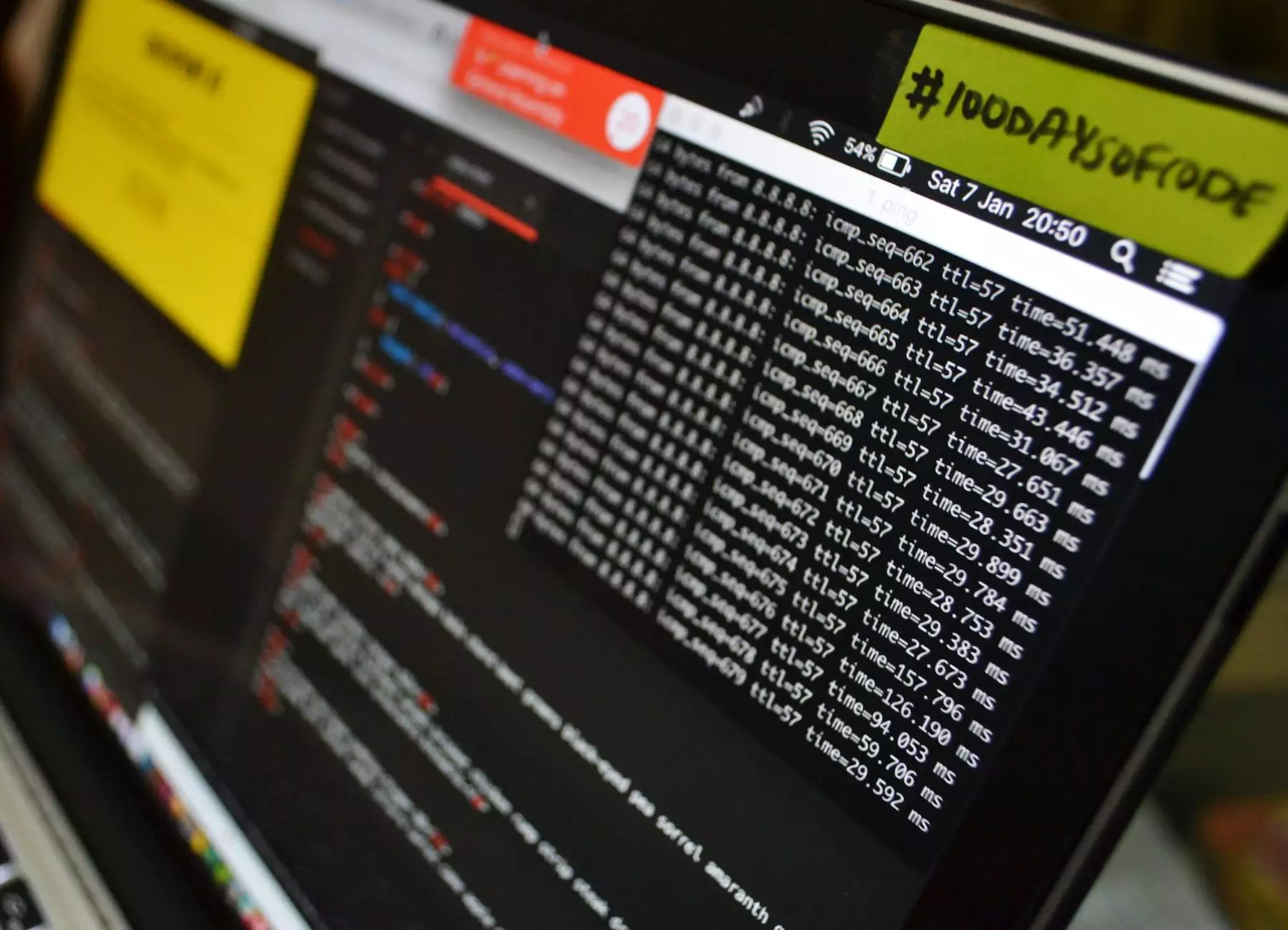
In the rapidly evolving landscape of technology and digital transformation, businesses that leverage high-quality, well-annotated data gain a decisive competitive advantage. Central to this is the process of data labeling, a critical component in training machine learning models and developing intelligent software systems. Whether it’s improving customer experiences, optimizing operations, or pioneering innovative products, effective data labeling is the backbone of modern software development strategies that propel businesses forward.
The Significance of Data Labeling in Today’s Business Environment
The growth of artificial intelligence (AI), machine learning (ML), and deep learning has revolutionized software applications across all industries. However, the success of these technologies hinges on the quality of data used during training. Data labeling transforms raw, unstructured data into meaningful, structured datasets that AI models can interpret, learn from, and act upon. High-quality labeled data enhances model accuracy, helps prevent errors, and ensures robustness — all vital for achieving business objectives.
Companies investing in advanced software development recognize that without meticulous data annotation, even the most sophisticated algorithms falter. Therefore, business success today increasingly depends on access to reliable data labeling solutions provided by specialized companies like Keymakr.
How Data Labeling Fuels Innovation in Software Development
Data labeling acts as the catalyst for innovation within the software development realm. By creating datasets that accurately represent real-world scenarios, businesses can train AI models to perform complex tasks such as image recognition, natural language understanding, speech processing, and predictive analytics. This, in turn, enables the development of cutting-edge applications such as autonomous vehicles, intelligent chatbots, personalized marketing systems, and healthcare diagnostics.
Furthermore, reliable data annotation supports innovation by reducing development cycles and increasing model reliability, which accelerates product deployment and adapts quickly to market needs. The role of data labeling is no longer optional but essential for businesses aiming to lead in a digital-first economy.
Business Value Derived from High-Quality Data Labeling
Investing in expert data labeling services provides measurable benefits:
- Enhanced Model Accuracy: Precise annotations ensure machine learning models learn correctly, reducing errors in predictions and classifications.
- Faster Time-to-Market: Efficient labeling workflows shorten development cycles, enabling faster rollout of AI-powered solutions.
- Cost Efficiency: Accurate data reduces the need for rework, saving resources and minimizing operational costs.
- Improved Customer Experience: Better data leads to more personalized, intuitive, and satisfying user interactions.
- Competitive Differentiation: Advanced AI capabilities derived from high-quality data distinguish market leaders from their competitors.
Key Categories of Data Labeling in Software Development
Various forms of data labeling are utilized depending on the application and data type:
- Image and Video Labeling: Annotating visual data for object detection, facial recognition, autonomous vehicle navigation, and surveillance systems.
- Text Annotation: Tagging entities, sentiments, keywords, and intents within textual datasets for natural language processing (NLP) applications.
- Audio Annotation: Labeling speech patterns, accents, language, and emotional tones for voice assistants and speech recognition systems.
- Sensor Data Labeling: Annotating data from IoT devices, wearables, or environmental sensors to enhance predictive analytics and automation.
- 3D Data Labeling: Annotating 3D point clouds and models used in robotics, AR/VR, and autonomous vehicles.
Each category demands specialized expertise to ensure annotations are accurate and contextually relevant, emphasizing the importance of partnering with experienced data labeling providers like Keymakr.
The Data Labeling Process in Software Development: A Step-by-Step Guide
Implementing effective data labeling involves several key stages designed to maximize accuracy and efficiency:
1. Data Collection and Preparation
Gathering vast amounts of raw data from various sources including images, videos, audio, and text. Ensuring data quality at this stage is crucial for subsequent labeling accuracy.
2. Data Review and Segmentation
Organizing data into manageable batches and performing initial quality checks to identify and discard unusable data.
3. Annotation and Labeling
Applying precise labels using specialized tools and annotation techniques. This step must adhere to strict guidelines to ensure consistency across datasets.
4. Quality Assurance and Validation
Implementing multi-tiered review processes, including manual checks by experts and automated validation tools, to confirm annotation accuracy.
5. Data Integration and Storage
Securely storing labeled data in structured formats compatible with machine learning pipelines, ready for training and deployment.
6. Continuous Improvement
Iteratively refining labeling guidelines and processes based on feedback and model performance metrics.
The Future of Data Labeling: Trends and Innovations Shaping Business Success
Emerging trends suggest that data labeling will continue to evolve, integrating more automation, semi-supervised learning, and crowdsourcing approaches. AI-powered labeling tools are becoming more sophisticated, reducing human effort while increasing precision.
Furthermore, the advent of synthetic data generation and simulation-based labeling offers exciting possibilities for creating vast, diverse datasets without extensive manual annotation. This innovation reduces costs and speeds up data collection for complex applications.
Businesses that stay ahead of these trends and adopt cutting-edge data labeling solutions can expect to see significant improvements in AI model performance, operational efficiency, and competitive positioning.
Why Leading Software Companies Choose Keymakr for Data Labeling
Partnering with Keymakr offers numerous advantages:
- Experience and Expertise: A team of skilled annotators familiar with diverse data types and industry standards.
- Customized Labeling Solutions: Tailored services aligned with your project goals, industry specifics, and data nuances.
- Scalable Workflows: Ability to handle large datasets efficiently, ensuring prompt project completion.
- Quality Control: Multi-layered quality assurance processes that maintain high annotation standards.
- Advanced Tools and Technologies: Cutting-edge annotation software that enhances productivity and accuracy.
- Data Security and Confidentiality: Robust measures to protect sensitive data throughout the labeling process.
Investing in a trusted data labeling partner like Keymakr ensures your software development efforts are built on reliable, high-quality data, ultimately leading to superior model performance and business results.
Conclusion: Data Labeling as a Critical Enabler of Business Innovation and Success
In an era where data-driven decision-making dominates, data labeling is more than just a technical task — it is a strategic imperative for forward-thinking businesses. By harnessing precise, scalable, and efficient data annotation processes, organizations unlock the full potential of AI and machine learning, creating smarter products, enhancing operational efficiency, and delivering unparalleled customer value.
Partnering with experienced data labeling providers such as Keymakr positions your business at the forefront of technological innovation, ensuring you stay competitive in an increasingly digital world.
Embrace high-quality data labeling today and transform your software development initiatives into powerful engines of growth and innovation.