Unlocking the Power of Image Labeling Tools for Object Detection
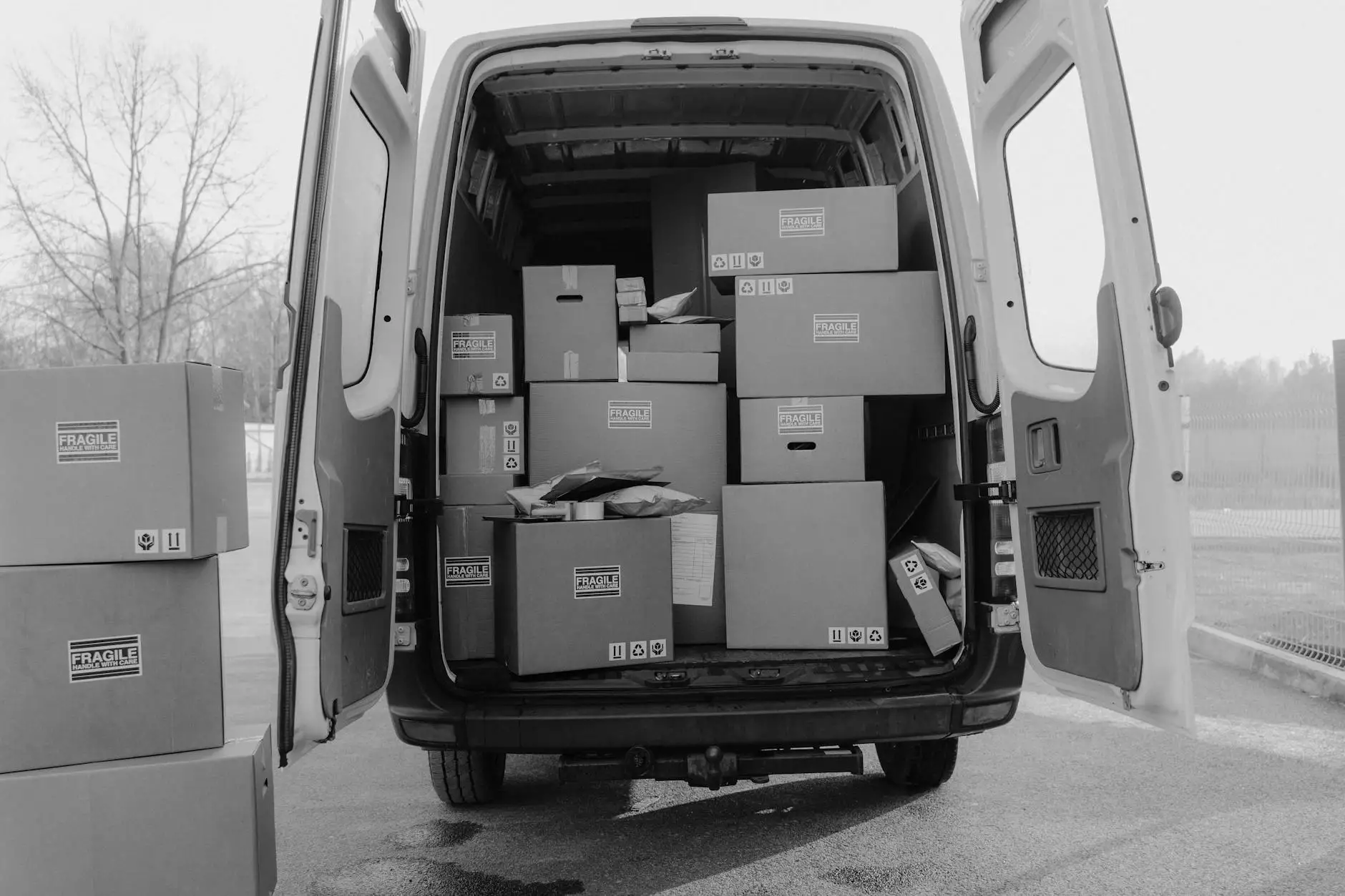
The rapid evolution of artificial intelligence (AI) has revolutionized the way we approach data processing and machine learning. One of the most crucial components of this evolution is image labeling tools for object detection, which enable machines to understand images effectively through data annotation.
What is Image Labeling?
Image labeling refers to the process of tagging images with metadata that describes the contents within them, facilitating machine learning projects. This process is essential for developing algorithms that can recognize objects within a scene. At the heart of object detection is the ability of AI systems to identify and locate various objects in images or videos. Image labeling tools play a pivotal role in training these systems.
The Importance of Data Annotation in Object Detection
Data annotation is crucial for enhancing the accuracy of machine learning models. By providing well-labeled datasets, data annotation ensures that models can learn effectively, leading to improved performance in tasks such as:
- Image Recognition: Identifying what objects are present in images.
- Object Localization: Pinpointing the location of objects within images.
- Segmentation: Understanding the boundaries of objects within an image.
Without high-quality labeled data, machine learning models may struggle to generalize from training datasets to real-world scenarios, reducing their effectiveness.
How Image Labeling Tools Work
Image labeling tools for object detection are designed to facilitate the annotation process efficiently. These tools allow users to:
- Upload images: Easily import images for labeling.
- Draw bounding boxes: Create boxes around objects for identification.
- Classify objects: Assign categories to different elements within the image.
- Export annotations: Save the labeled data in various formats compatible with machine learning frameworks.
Each of these features contributes significantly to streamlining the data preparation phase, which is often the most time-consuming aspect of training an AI model.
Key Features of Effective Image Labeling Tools
When selecting an image labeling tool for object detection, it's vital to consider several features that can greatly impact your workflow:
- User-Friendly Interface: A straightforward interface allows users to navigate the tool with ease, saving precious time.
- Customizable Labeling Options: Flexibility in labeling styles and options ensures that the tool can adapt to various project needs.
- Collaboration Features: Efficient workflows require collaboration. Look for tools that enable multiple users to work simultaneously.
- Quality Control Mechanisms: Built-in quality checks can help maintain labeling accuracy and consistency.
- Integration Capabilities: Opt for tools that can integrate seamlessly with existing data pipelines and machine learning frameworks.
These features reflect the effectiveness and efficiency of image labeling tools in enhancing the data annotation process.
Popular Image Labeling Tools for Object Detection
There are several image labeling tools for object detection available in the market today, each offering unique features and capabilities. Here are some of the most recognized tools:
- LabelBox: A versatile platform that provides a wide range of annotation tools, LabelBox excels in user collaboration and automating workflows.
- Supervisely: Known for its comprehensive feature set, Supervisely supports image and video annotation, making it ideal for complex projects.
- VGG Image Annotator (VIA): A free, open-source tool, VIA is straightforward and easy to use for smaller projects and educational purposes.
- RectLabel: Particularly useful for Mac users, RectLabel offers robust image annotation capabilities with easy export options.
- Keylabs.ai: A cutting-edge platform that simplifies the data annotation process with excellent quality control and collaboration tools.
Each of these tools has its strengths, and the choice often depends on specific project requirements and team dynamics.
Best Practices for Effective Image Labeling
To achieve the best results from image labeling, consider the following best practices:
- Define Clear Guidelines: Establish definition and categories to ensure consistent labeling across your team.
- Utilize Automation: Leverage any AI-assisted features within your labeling tools to speed up the process and maintain accuracy.
- Regular Reviews: Implement periodic audits of labeled data to check for errors and maintain a high quality.
- Train Your Team: Ensure all team members are adequately trained on how to use the tools and follow the labeling guidelines.
Following these best practices can significantly enhance the quality and reliability of your data annotation efforts.
Conclusion
In conclusion, image labeling tools for object detection are indispensable in today’s data-driven landscape. They empower AI frameworks to learn from visual inputs accurately, which is critical in applications ranging from autonomous vehicles to medical diagnosis. Platforms like Keylabs.ai not only streamline the data annotation process but also enhance collaboration among teams, improving the overall efficiency of machine learning projects.
By understanding the features and benefits of various tools and adopting best practices, businesses can leverage the full potential of data annotation to drive innovation and efficiency in their AI initiatives. As we continue to explore the possibilities of AI, investing in high-quality image labeling tools will be paramount to success.